Data Attribution in Ecommerce: What Makes Swanky’s Approach Unique?
Navigating data attribution in ecommerce can be confusing and lead to misinformed decisions. Learn from Tom Cox, Data & Analytics Manager at Swanky, about how Swanky’s approach leads to clarity and growth.
Written By
Tom Cox
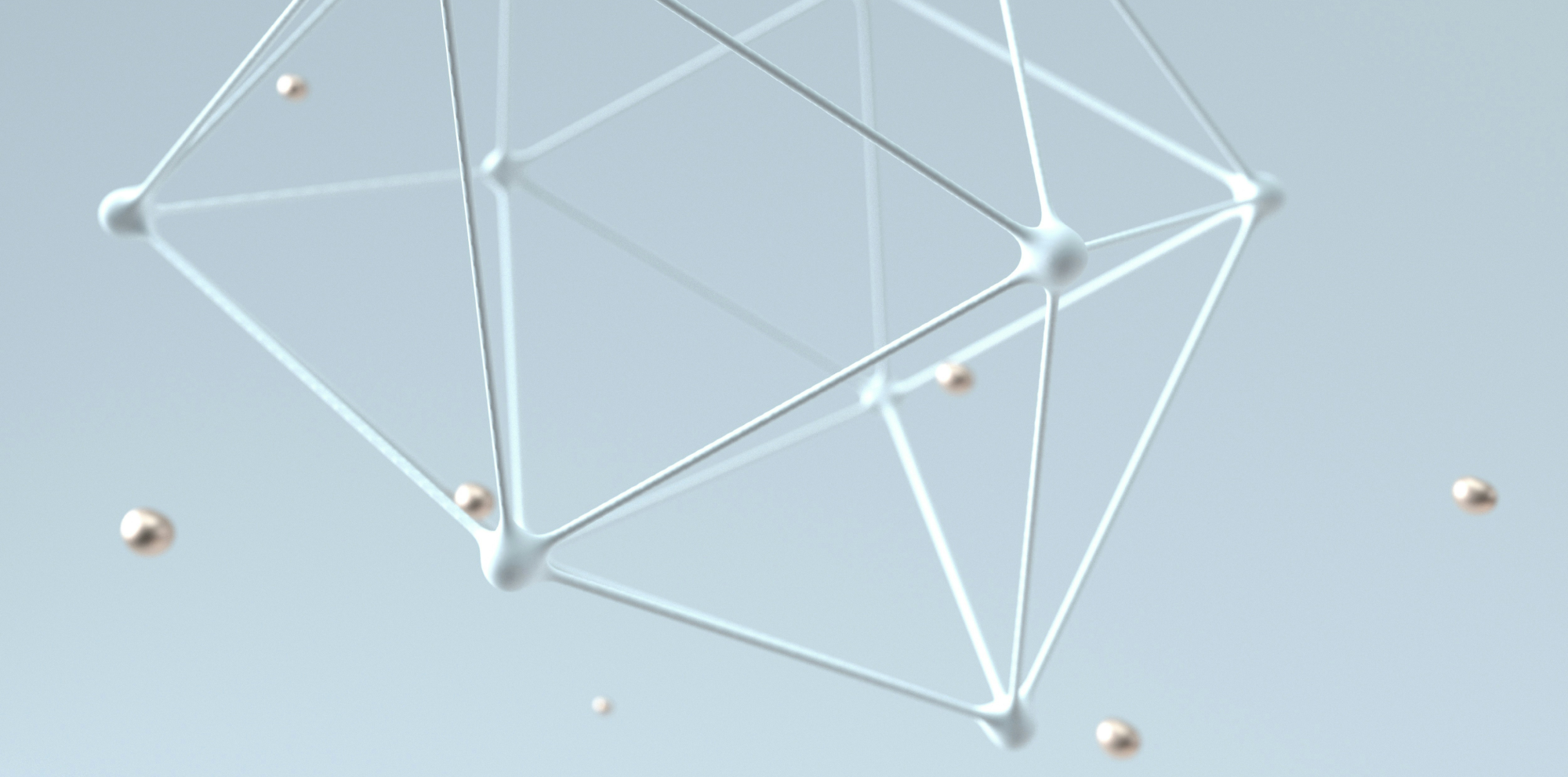
It’s widely agreed that data is a foundational element of any ecommerce store, yet the process of data attribution can create confusion amongst teams.
Data attribution models are designed to give clarity and structure to your store’s statistics. However, there are numerous models you can use and it can be hard to know which is best suited for your brand.
Here at Swanky, we’ve developed an approach to data attribution that aims to enhance clarity, increase trust and lead to data-informed decision-making.
What is a data attribution model?
Data attribution models allow us to better understand which online marketing channels contributed to particular actions. This could be anything from signing up to an email list, booking an appointment or making a purchase.
Over the past decade, it’s become a requirement of marketing teams worldwide to evidence how users are interacting with marketing and accessing an ecommerce store.
Users will rarely make a purchase on their first visit to a site. Instead, they will typically engage with a site across several different sessions. These can be a combination of organic searches, social and PPC retargeting, and email abandonment flows.
Attribution is an attempt to understand which channels are contributing to conversions.
How does data attribution work?
Data attribution models often rely on a combination of first-party cookies, UTM parameters and tracking scripts to capture as much session and event data as possible. Through using this data, we can build a picture that shows:
- how users arrive at a website;
- how retargeting is working; and
- how engaging email campaigns and flows are.
Choosing which model to use is important and depends on your brand, the marketing channels that you are using and the types of campaigns that you are running.
Outlined below are four different models for you to consider.
First Click / First Touch
The First Click / First Touch model attributes the revenue of a transaction to the first touchpoint that brought the user to the site. Such a model is good for analysing the performance of brand awareness and Top of Funnel (TOF) strategies.
This model is great to decipher which campaigns are most effective for acquiring new potential customers who aren’t yet aware of your brand, or are in the research phase of their buying process.
Last Click
Last Click is a great model for pinning down which campaigns were the final push that led to a customer making a purchase. This is a good approach for analysing how retargeting, flows or abandonment campaigns are performing.
We often use this model during sale seasons when we are considering a short window of time for click attribution. This helps ensure that the relevant email campaign or targeted promotional ad gets the credit for the conversion.
Linear
Linear based models will evenly distribute the conversion value between each of the different sessions that led to a conversion.
This is more useful for brands selling high price-point items, where the purchase journey is often longer, or for brands that leverage a wide range of marketing methods. A linear model allows credit to be attributed correctly to a range of campaigns, whether aiming for brand awareness or the final nudge towards completing checkout.
Data-driven
Data-driven attribution models are becoming more common across platforms. Both GA4 and Google Ads now utilise data-driven attribution as the standard default model.
Whilst there is no official documentation on exactly how Google uses this model, we know that it does so based on the quality of each session. This process uses machine learning and advanced analytics to assign revenue to different channels.
GA4 applies this model to assign fractional conversions to different sources based on the user journey that led to a conversion. It is also possible to view the different touchpoints of a customer journey in GA4 and which of these are being assigned credit.
Model comparison report in GA4
Conversion report in GA4
What alternatives are there to data attribution?
Marketing Mix Modeling
Marketing Mix Modeling (MMM) is becoming popular in all industries, particularly as tracking across the web continues to get harder. This is based on statistical modelling that evaluates the spend across all platforms (including radio, TV advertising, direct mail and other traditional media methods).
When MMM is set up, it indicates how each marketing channel contributes to overall revenue. This gives decision makers a better idea of where to invest their marketing budget.
In the past, MMM has typically been reserved for enterprise setups. This is due to the fact that, historically, MMM required a substantial dataset, collected over several years, and data scientists to create and maintain the model. However, in March 2024 Google announced the upcoming release of Meridian, an open-source MMM that would make this data attribution model more broadly accessible by reducing the costs previously associated with it.
How does Swanky approach data attribution in ecommerce?
Data attribution relies on good quality data and meticulous maintenance of ad platform setups and pixels. Therefore, the priority for Swanky has always been to capture the best data possible from every area of a store, ensuring that Shopify, GA4 and other platforms have comprehensive datasets to draw from.
In practice, we always carry out a thorough data audit before beginning any project. This ensures conversion events are correctly set up (ideally using a server-side, first-party solution) and that pixels are installed accurately. We also review a client’s UTM strategy to ensure no data is being lost along the way.
The result of this process is transparency of data and performance. This establishes a strong foundation for success and offers us the flexibility to apply the most suitable attribution model according to the marketing channels in use.
With this foundation in place we can accurately measure campaign performance. We can then confidently assign and encourage investment in successful areas, whilst reducing spend for less effective campaigns.
Why is our approach best-in-class?
Our approach allows us to strategically deploy multi-channel marketing campaigns, based on data-driven decision making. This is only possible with full visibility of each channel’s contribution to a conversion and leads to consistently high-performing campaigns across email, Google Ads, Meta and others.
Swanky’s approach to data attribution is unique. Our competitors will typically spend time siloed within a single platform, optimising and investing in advertising based on unreliable platform data. This is often powered by a client-side tracking solution which increases the likelihood of errors and incorrect data.
We opt to use first-party, server-side solutions for our clients, and we continually update our tags in accordance with the latest industry standards. Our marketing teams can be confident they are getting the best possible value for each dollar spent on advertising. This confidence translates to cohesive and data-driven marketing campaigns.
Swanky’s data tech stack
The ecommerce industry continues to innovate and improve the options available for data attribution. Most platforms now have attribution functionality built into them, allowing you to view different attribution models with a single click. However not all of these provide the quality or type of data that drives ecommerce growth.
Polar Analytics
Swanky has partnered with Polar Analytics as our preferred analytics platform. Polar has created a one-click solution that instals a first-party pixel on your Shopify site and provides access to many different models.
We are acutely aware of the tendency for a platform to over emphasise its attribution efforts, particularly Meta. For this reason, having another source of information where we can compare revenue, ROAS (return on ad spend) and CAC (customer acquisition cost) for all marketing channels, provides the highest level of transparency for our team and clients.
Model comparison report in Polar Analytics.
Littledata
We also work closely with Littledata: a tool that provides a server-side tracking solution that pushes the best quality data into organic social and PPC channels. This not only provides more accurate reporting within each platform, but also improves the quality of campaigns that engage enhanced datasets (such as Performance Max for Google Ads and Advantage+ for Meta).
Moreover, we’ve found that Littledata provides the most comprehensive GA4 implementation solution within the Shopify ecosystem – with installation taking less than 15 minutes.
Using Littledata, we are able to confidently utilise GA4’s reporting to better understand which channels are driving conversions.
Learn more about Swanky’s data services
Data is a vital part of ecommerce growth. It can also be overwhelming. If you’re serious about scaling your ecommerce brand, we recommend making sure your data setup is match-ready.
If you’re not confident in your data setup, consider getting a data audit with our team. This will empower you to trust the foundation of your site and make informed, data-driven decisions when it comes to resourcing and strategy.
Get in touch today to begin the conversation.